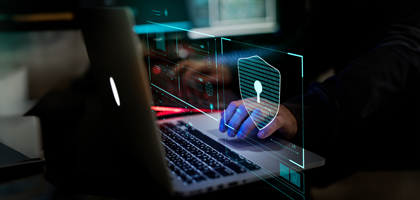
Insights
Advancing Healthcare Innovation with Privacy at the Forefront
The General Data Protection Regulation (GDPR) is a European Union regulation since May 25, 2018, on information privacy in the European Union and the European Economic Area. The GDPR is an essential component of EU privacy law and human rights law, particularly Article 8 of the Charter of Fundamental Rights of the European Union.
It is the strictest privacy and security law in the world. It establishes principles and rules for the lawful and fair processing of personal data, giving individuals greater control over it. It applies not only to organizations based in the EU but also to those outside the EU that process the personal data of EU residents. One of the main focuses of the GDPR is protecting personal data.
Read More...
But What Is Personal Data?
As is defined in Article 4 (1), personal data is any information that is related to an identified or identifiable natural person. In other words, different pieces of information are collected and can lead to identifying a particular person. In addition to general personal data, one must consider all the special categories of personal data (also known as sensitive personal data) above. These data include genetic, biometric, and health data, as well as personal data relating to race, ethnic origin, political opinion, philosophical belief, religion, religious sect or other belief, appearance, membership to associations, foundations, or trade unions, data concerning health, sexual life, criminal convictions, and security measures.
KVKK (Kişisel Verilerin Korunması Kanunu - Personal Data Protection Law) is the Turkish counterpart to the GDPR, governing the protection of personal data in Turkey. However, KVKK applies explicitly within the borders of the Republic of Türkiye. GDPR and KVKK share common principles, as they are designed to ensure the responsible and lawful processing of personal data, respect for individuals' privacy rights and implement any necessary security measures to protect this type of data.
At Tiga Healthcare Technologies, we develop innovative data-driven projects in healthcare by leveraging cutting-edge technology in compliance with these regulations. For a couple of our projects, such as AISym4MED (EU project) and Autononym, where we are dealing with susceptible data, we are committed to the highest standards of data protection and privacy while ensuring the confidentiality and security of confidential information in collecting, storing, transferring and processing phases.
Although there is a vast amount of stored health data, accessibility is hindered by several issues, such as privacy and anonymization concerns. Therefore, our utmost priority is to safeguard against privacy breaches, adhering to ethical and legal standards. Building trust between Tiga Healthcare Technologies and its customers is crucial for fostering long-term relationships and ensuring the long-term sustainability of our business to gain a competitive edge. We want to present some measures to address these concerns while working on these projects.
Anonymization is a process of data obfuscation that breaks associations between data and the individual to whom the data was attributable. Adequate anonymization plays a crucial role in healthcare, facilitating the responsible sharing of data for research and analysis with numerous potential benefits. Anonymization is grounded on two fundamental pillars.
The first pillar, ‘Masking,’ dramatically reduces the risk of identifying a data subject by employing transformative techniques that render direct identifiers such as names and Social Security numbers virtually untraceable. Masking significantly distorts the data so that no analytics can be performed. However, analytics on masked fields are typically unnecessary. For example, for a name column in the dataset, ‘John Doe’ can be masked into ‘Patient_1’, ‘Jane Smith’ can be hidden into ‘Patient_2’ and so on.
The second pillar, ‘De-identification,’ achieves a similarly low risk of identification but focuses on preserving high analytic utility. This involves safeguarding fields related to demographics and socio-economic information, including age, home and work ZIP codes, income, number of children, and race, which are considered indirect identifiers. De-identification strategically minimizes data distortion, allowing meaningful analytics while upholding credible privacy protection claims. Thus, de-identification strives to strike a delicate balance between data utility and privacy considerations. To give an example, for two columns ‘City’ and ‘State’ existing in the dataset, such as ‘City_A, State_X,’ ‘City_B, State_Y’ and ‘City_C, State_Z,’ the de-identification process involves aggregating specific geographic details (City and State) into broader regions. The original, potentially identifying information of an individual is replaced with a more generalized descriptor (e.g., ‘Region_1,’ ‘Region_2,’ ‘Region_3’). This helps protect individual privacy by reducing the granularity of geographic data while still preserving the overall regional context. De-identification in this context allows for meaningful healthcare data analysis without exposing precise geographical details.
Additionally, in addressing the paramount concern of safeguarding the privacy of healthcare data, our company has embraced another popular approach — the generation of synthetic health data, which has emerged as a promising method as a privacy-conscious alternative to raw data methods while sharing individual-level health data in a privacy-preserving manner.
The Evaluation of The Synthetic Data
Algorithmically generated, synthetic data replicates statistical properties and patterns observed in the source data (original data). But importantly, it contains no sensitive, private, or personal data points. The evaluation of the synthetic data revolves around three essential dimensions: (1) Fidelity, (2) Utility, and (3) Privacy.
Fidelity
Fidelity refers to the degree of similarity between synthetic data and the original, real-world data. High fidelity ensures that the synthetic data effectively mirrors the source data's statistical properties, patterns, and structures. It is a measure of how well the generated data captures the essence of the original dataset. Fidelity evaluation can be done in a couple of approaches: exploratory statistical comparisons, histogram similarity scores, mutual information, correlation/autocorrelation, and partial autocorrelation scores.
Utility
After establishing the statistical similarity between the synthetic and original datasets, evaluating the synthesized dataset's performance across everyday data science challenges is imperative when training various machine learning algorithms. This is where utility comes into play, determining the practical effectiveness of synthetic data for a specific purpose or task. Beyond maintaining fidelity, a synthetic dataset must prove its applicability and practicality for its intended use, whether in algorithm testing, model training, or other analytical and developmental processes. By employing utility metrics, we aim to instill confidence in our ability to replicate the performance of the original data in downstream applications. There are a couple of metrics proposed in the literature to measure the utility, such as prediction scores (Train on Real, Test on Synthetic (TRTS), Train on Real, Test on Real (TRTR), Train on Synthetic, Test on Real (TSTR), and Train on Synthetic, Test on Synthetic (TSTS) scores), QScore and feature importance score.
Privacy
Privacy in the context of synthetic data refers to protecting sensitive, private, or personally identifiable information. Synthetic data generation aims to eliminate any direct connection to actual individuals or sensitive details. However, before sharing freely and using for downstream applications, the residual privacy risks must be ex-post assessed to prevent potential leakage. This assessment uses privacy metrics regarding the extent of leaked information. These metrics include but are not limited to exact match score, neighbors' privacy score, membership inference score and such.
In our relentless pursuit of privacy-centric data innovation, we recognize that the evolving healthcare landscape demands a multifaceted approach. In addition to the robust measures of anonymization, de-identification, and synthetic data generation, we remain vigilant and explore emerging techniques that further fortify privacy safeguards. Differential privacy, for instance, stands out as a promising avenue, introducing noise to individual data points to protect against re-identification risks. Homomorphic encryption, another frontier, enables secure computation on encrypted data, fostering collaboration without compromising sensitive information. These evolving methodologies and our current arsenal of privacy-preserving strategies exemplify our dedication to staying at the forefront of data protection. By embracing a diverse toolkit of privacy-enhancing technologies, we adapt to the ever-changing landscape of data privacy and reinforce our commitment to pioneering ethical, responsible, and innovative healthcare solutions.
At Tiga Healthcare Technologies, we believe that fostering trust, respecting privacy, and upholding the highest data protection standards are not just obligations but integral components of our mission to revolutionize healthcare through cutting-edge, responsible, data-driven projects.